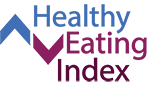
On this page...
This web page describes the methods that have been developed for use with the HEI and discusses some of the limitations of each method. The methods are applicable to both the HEI (for 2 years and older) and the HEI-Toddlers-2020 (for 12 through 23 months). There are two categories of methods described below; one category includes methods that do not estimate usual intake. The second category describes methods that have been developed to estimate usual dietary intake. Selectin a method will depend on the purpose of your research and the data you have available, although the HEI can be applied to 24 hour recalls (24HR), food records, and food frequency questionnaires (See Table 1 below). Note also that the methods described below were developed first with 24HR data for population monitoring research.
Table 1: Dietary Assessment Tools, Study Purpose and the HEI
Recommended methods to calculate Healthy Eating Index scores depending on the main purpose of the study
Main Purpose of the Study | 24 Hour Recall1 | Food Frequency Questionnaire |
---|---|---|
Group of Individuals | ||
Describing mean diet quality among a population | Population ratio method recommended as a less biased measure of usual HEI scores (single recall or record administration required) Bivariate method recommended if replicate recalls available for at least a subsample, but more computationally intensive Means estimated using the person-level algorithm may be acceptable if interest is in a specific day(s) |
FFQ data not recommended for this purpose due to biases |
Estimating distributions of diet quality among a population | Multivariate method recommended for total and component scores; accounts for interrelationships among components Bivariate method possible for components scores; does not account for interrelationships among components |
FFQ data not recommended for this purpose due to biases |
Examining associations between diet quality and a dependent variable | Person-level scores may be acceptable if interest is in a specific day(s) | Person-level score possible but biases associated with reporting error and characteristics of the FFQ (e.g., coverage of the foods typically consumed by the individual) should be considered Other methods incorporating FFQ data are under development |
Examining associations between an independent variable and diet quality | Person-level scores may be acceptable if interest is in a specific day(s) | Person-level score possible but biases associated with reporting error and characteristics of the FFQ (e.g., coverage of the foods typically consumed by the individual) should be considered Other methods incorporating FFQ data and 24 HR data are under development |
Assessing the effects of interventions on diet quality | Population ratio method (with standard errors) can be used to compare scores among groups Bivariate and multivariate methods could be used to compare component and total scores, respectively, among groups |
Person-level score possible but biases associated with reporting error and characteristics of the FFQ (e.g., coverage of the foods typically consumed by the individual) should be considered Other methods incorporating FFQ data and 24 HR data are under development |
Individuals | ||
Describing diet quality for an individual within a clinical setting2 | Person-level score possible; biases associated with reporting error should be considered; due to day-to-day variation in intake, many recalls are needed to capture usual diet quality | Person-level score possible but biases associated with reporting error and characteristics of the FFQ (e.g., coverage of the foods typically consumed by the individual) should be considered |
1 Methods applicable to 24-hour recalls may also be applicable to data from food records/diaries.
2 A Personalized Healthy Eating Index Nutrition Report is under development. This report can be used in clinical practice and individual nutrition counseling.
Methods Not Estimating Usual Intake
The simple HEI scoring algorithm method is applied to calculate scores using computed amounts of each component in the HEI. To use the simple HEI scoring method, first the ratio of the dietary constituent to energy is constructed and scored according to the scoring standards. The component scores are summed to calculate the total score. The mean total score is the mean of the total scores across individuals. When more than one 24HR per person is available, the score is calculated by summing across all days per person before scoring.
In the mean ratio method, as in the simple HEI scoring algorithm method, first compute the ratio of each dietary constituent to energy. However, rather than scoring these ratios for each individual as in the simple scoring algorithm method, the means of the ratios over individuals are then computed. Finally, the HEI component score is calculated using the mean ratio for each component, and the total score is calculated by summing the scores over the components. When more than one 24HR per person is available, the score is calculated in the same manner, using both recalls for a participant.
The population ratio method is used to calculate the mean intakes of dietary constituents, and scoring standards are applied to arrive at scores at the level of a group of persons. To apply the population ratio method, the intake of the relevant dietary constituents and energy are summed for all individuals in a population to obtain estimates of the population’s total intake, and then the ratios of each constituent to energy are computed and scored. The total score is then the sum of the component scores. While this method does not estimate usual intake at the individual level, it may be used to estimate usual intake at the population level.
Methods Estimating Usual Intake
With two or more recalls per person (on at least a subset of individuals), methods that distinguish day-to-day variation from variation between individuals (“usual intake methods”) may be used. Two usual intake methods have been developed: the bivariate method is a computational modeling approach that is used to simultaneously model two dietary constituents. This creates predicted ratios and the application of scoring standards to predict component and total scores, as well as distributions of component scores. Note that although this method allows estimation of distributions of component scores, estimation of the distribution of total scores requires a multivariate approach, as described below. The bivariate method uses the NCI Method measurement error methodology to jointly estimate usual intake for a food or nutrient dietary constituent and energy, accounting for the correlation between the two dietary constituents (Freedman et al., 2010). This method can be used to estimate each component of the HEI score.
The multivariate Markov Chain Monte Carlo (MCMC) approach is similar to the bivariate approach, but extends the methodology to jointly model all components of the HEI simultaneously, accounting for the correlation among all components. The multivariate MCMC method requires that none of the model variables include a subset of the other. Therefore, constituents such as legumes must be modeled separately and added together prior to scoring.
Distributions and the Usual Intake Methods
Both usual intake methods are an extension of the two-part model used in the NCI Method (Tooze et al., 2006), which can be used to estimate distributions of usual intake for both nutrients and foods, whether episodically-consumed or not. The first part models the probability of consuming a food on a particular day, and the second part models the consumption-day amount. The bivariate method extends this model by adding a third part – a model for the amount of energy consumed on a given day. Maximum likelihood may be used to fit this 3-part model. The multivariate model extends it to a multi-part model, which is comprised of 2 parts for each episodically-consumed component, an amount-only model for each constituent consumed daily, plus energy (details of the exact dietary constituents modeled are described in the Multivariate MCMC Steps below) (Zhang et al., 2011). Due to the complexity of this model, an approach called Markov Chain Monte Carlo (MCMC) is used to fit the model rather than maximum likelihood.
To obtain the distribution of usual intake from either usual intake method, the input from the model is used in a Monte Carlo step to generate ratios. This is a simulation step in which a number of “pseudo-individuals” are generated for each actual person in the dataset, using the estimated model parameters to simulate realizations of the distribution for each pseudo-individual. Next, the ratio of usual dietary component intake to energy intake is calculated for each pseudo-individual and the HEI score is calculated from this ratio. The mean and percentiles of the distribution of the HEI components scores and total scores are then calculated to obtain estimates for the distribution.
Limitations and Considerations
Each method described here has limitations to its use in research. Some of the limitations are described below. For example, some of the methods do not consistently address measurement error, deal with episodic intake, adjust for skewness, or appropriately model intake given the correlation between the components energy, resulting in different effects on the scores. In contrast, the MCMC method does address measurement error, episodic consumption, skewness, and the correlation between the components and energy. For a comparison of the limitations of the various methods, see Table 2.
Table 2. Comparison of the Limitations of Methods
Simple | Mean Ratio | Population Ratio | Bivariate | MCMC | |
---|---|---|---|---|---|
Limitations | |||||
Adjusts for Measurement Error | No | Using mean | Using mean | Yes, model | Yes, model |
Considers Episodic Consumption | No | Yes, but ignores correlation between probability and amount | Yes, but ignores correlation between probability and amount | Yes | Yes |
Considers Skewness | No | No | No | Yes | Yes |
Accounts for Correlation Between Each Constituent and Energy | Yes, individual level | No | No | Yes | Yes |
Accounts for Correlation Between All Constituents and Energy | No | No | No | No | Yes |
Limitations to using the HEI and limitations on the methods used to calculate the score come from a variety of sources. Diet assessment tools used, number of recalls or records collected, the variability of day-to-day diets and the fact that some dietary components are correlated with others all play a role in the limitations discussed here. Understanding some of the limitations involves understanding concepts such as measurement error, episodic intake, skewness, and correlation. Click on the links below for a more in-depth look at some of the topics.
- Measurement error refers to the difference between the observed or measured value and the true value. Self-report dietary assessment instruments are affected by two main types of error - systematic and random (within-person random error) - that must be understood and addressed to avoid misleading results.
- Episodic intake refers to the fact that some nutrients and foods are not consumed daily by nearly everyone in the population, so intake may be reported as zero on a particular day. Other dietary constituents are not episodic and are consumed daily by nearly everyone in the population, so intake is rarely reported as zero on a particular day.
- Skewness refers to dietary data that are not normally distributed, rather they are skewed to the left or right. For example, fruit is frequently reported in small quantities by many people, though for people who do consume fruit, they typically consume it in substantial quantities. This results in a distribution that is skewed to the right.
- Correlation is a measure of linear association of two variables. In dietary data, variables are often correlated with each other or with overall energy intake.
Another limitation is the inherent day-to-day variability in the human diet. With only one day of intake, the mean but not the distribution of usual intake in the population can be estimated. A single day of intake does not represent the usual intake of an individual, so when the simple HEI scoring algorithm is applied to one day, day-to-day variability is included in the estimate of the HEI, leading to a great deal of noise. Both the mean ratio method and the population ratio method adjust for day-to-day variation by averaging recalls across the population to arrive at a score that is closer to usual intake. The dietary intake ratios that are derived using these methods therefore are adjusted for day-to-day variation across individuals, but not within an individual. Estimates of both within-person and between-person variation are needed to estimate the distribution of usual intake.
Both usual intake methods account for measurement error due to day-to-day variability in intake, as described above. Both also account for skewness of dietary data by using a Box-Cox transformation of the consumption day amount data in the model. Under normality, the mean and median are the same, and therefore “average” intake is easier to interpret and understand. When data are skewed right, the mean will be larger than the median, and the measure of the mean as the measure of central tendency is harder to comprehend. Both the bivariate and multivariate methods use regression models, so they may also adjust for weekend/weekday or sequence effects of the 24HRs, and they may include subpopulation covariates (e.g., age group) to obtain subpopulation estimates.
References
Freedman LS, Guenther PM, Krebs-Smith SM, Dodd KW, Midthune D. A population's distribution of Healthy Eating Index-2005 component scores can be estimated when more than one 24-hour recall is available. J Nutr. 2010 Aug;140(8):1529-34.
Tooze JA, Midthune D, Dodd KW, Freedman LS, Krebs-Smith SM, Subar AF, Guenther PM, Carroll RJ, Kipnis V. A new statistical method for estimating the usual intake of episodically consumed foods with application to their distribution. J Am Diet Nutr. 2006 Oct;106(10):1575-87.
Zhang S, Midthune D, Guenther P, Krebs-Smith SM, Kipnis V, Dodd KW, Buckman DW, Tooze JA, Freedman L, Carroll RJ. A new multivariate measurement error model with zero-inflated dietary data, and its application to dietary assessment. Ann Appl Stat. 2011 Jun 1;5(2B):1456-1487.